Registration Desk: Registration / Badge Pickup Tue 18 Jun 07:00 a.m.
The 3rd Workshop on Transformers for Vision Tue 18 Jun 07:50 a.m.
Workshop: Agriculture-Vision: Challenges & Opportunities for Computer Vision in Agriculture Tue 18 Jun 08:00 a.m.
Workshop: VizWiz Grand Challenge: Describing Images and Videos Taken by Blind People Tue 18 Jun 08:00 a.m.
Computer Vision for Materials Science Workshop Tue 18 Jun 08:10 a.m.
Workshop: 2nd Face Recognition Challenge in the Era of Synthetic Data (FRCSyn) Tue 18 Jun 08:10 a.m.
Workshop: The Future of Generative Visual Art Tue 18 Jun 08:20 a.m.
Workshop: Women in Computer Vision Tue 18 Jun 08:30 a.m.
Tutorial: Naoki Wake · Zane Durante · Ran Gong · Jae Sung Park · Bidipta Sarkar · Rohan Taori · Yusuke Noda · Yejin Choi · Demetri Terzopoulos · Katsushi Ikeuchi · Hoi Vo · Li Fei-Fei · Jianfeng Gao · Qiuyuan Huang
Generalist Agent AI (GAA) is a family of systems that generate effective actions in an environment based on the understanding of multimodal sensory input. While these systems are expanding into various fields with the advent of large foundation models, they share common interests such as data collection, benchmarking, and ethical perspectives. In this tutorial, we focus on several representative research areas of GAA, including gaming, robotics, and healthcare, and aim to provide comprehensive knowledge on the common concerns discussed in these fields. We expect the participants to learn the fundamentals of GAA and gain insights to further advance their research. Specific learning outcomes include: - GAA Overview: A deep dive into its principles and roles in contemporary applications, providing attendees with a thorough grasp of its importance and uses. - Methodologies: Detailed examples of how LLMs and VLMs enhance GAAs, illustrated through case studies. - Performance Evaluation: Guidance on the assessment of GAAs with relevant datasets. - Ethical Considerations: A discussion on the societal impacts and ethical challenges of deploying Agent AI, highlighting responsible development practices. - Future Challenges: A categorization of the latest developments in each domain and a discussion of future directions. Led by experts from academia and industry, we expect the tutorial to be an interactive and enriching experience. This event will include talks, Q&A sessions, and a panel discussion, ensuring a comprehensive and engaging learning experience for all participants.
Bio s: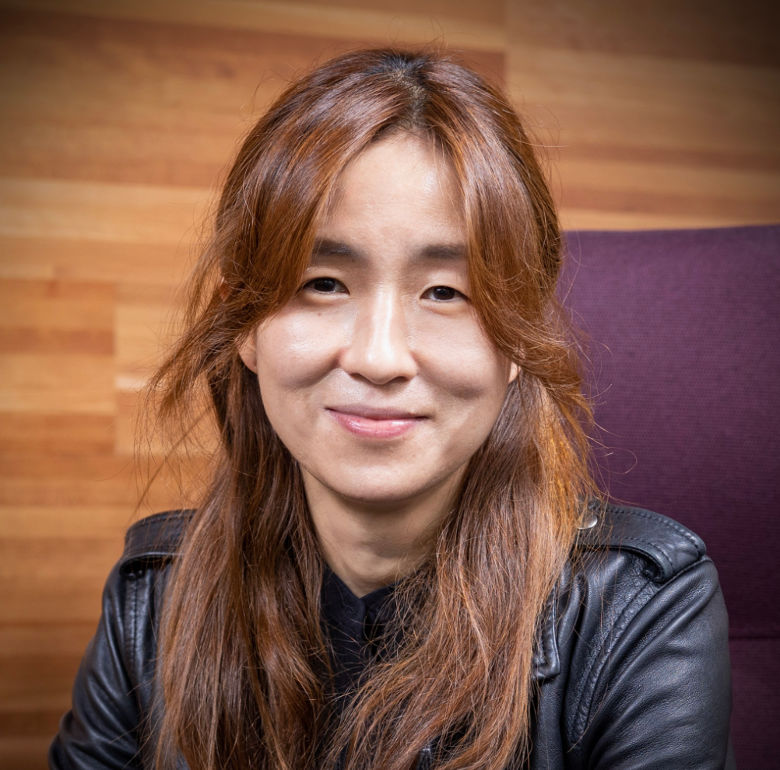
10th IEEE International Workshop on Computer Vision in Sports (CVsports) Tue 18 Jun 08:30 a.m.
ReGenAI: First Workshop on Responsible Generative AI Tue 18 Jun 08:30 a.m.
LatinX in Computer Vision Research Workshop Tue 18 Jun 08:30 a.m.
Workshop: RetailVision - Field Overview and Amazon Deep Dive Tue 18 Jun 08:30 a.m.
Workshop on Responsible Data Tue 18 Jun 08:30 a.m.
1st Workshop on Test-Time Adaptation: Model, Adapt Thyself! (MAT) Tue 18 Jun 08:30 a.m.
2nd Workshop on What is Next in Multimodal Foundation Models?'' Tue 18 Jun 08:30 a.m.
Third Workshop of Mobile Intelligent Photography & Imaging Tue 18 Jun 08:30 a.m.
This workshop focuses on Mobile Intelligent and Photography Imaging (MIPI). It is closely connected to the impressive advancements of computational photography and imaging on mobile platforms (e.g., phones, AR/VR devices, and automatic cars), especially with the explosive growth of new image sensors and camera systems. Currently, the demand for developing and perfecting advanced image sensors and camera systems is rising rapidly. Meanwhile, new sensors and camera systems present interesting and novel research problems to the community. Moreover, the limited computing resources on mobile devices further compound the challenges, as it requires developing lightweight and efficient algorithms. However, the lack of high-quality data for research and the rare opportunity for an in-depth exchange of views from industry and academia constrain the development of mobile intelligent photography and imaging.
With the consecutive success of the 1st MIPI Workshop@ECCV 2022 and the 2nd MIPI Workshop@CVPR 2023, we will continue to arrange new sensors and imaging systems-related competition with industry-level data, and invite keynote speakers from both industry and academia to fuse the synergy. In this MIPI workshop, the competition will include three tracks: few-shot raw denoising, event-based sensor, and Nighttime Flare Removal. MIPI wishes to gather researchers and engineers together, encompassing the challenging issues and shaping future technologies in the related research directions.
Workshop: Computer Vision with Humans in the Loop Tue 18 Jun 08:30 a.m.
GAZE 2024: The 6th International Workshop on Gaze Estimation and Prediction in the Wild Tue 18 Jun 08:30 a.m.
Workshop: What is Next in Video Understanding? Tue 18 Jun 08:30 a.m.
9th Workshop on Computer Vision for Microscopy Image Analysis Tue 18 Jun 08:30 a.m.
XRNeRF: Second Workshop on Advances in Radiance Fields for the Metaverse Tue 18 Jun 08:30 a.m.
The 7th Workshop on Efficient Deep Learning for Computer Vision Tue 18 Jun 08:30 a.m.
Tutorial: Samet Akcay · Paula Ramos Giraldo · Ria Cheruvu · Alexander Kozlov · Zhen Zhao · Zhuo Wu · Raymond Lo · Yury Gorbachev
This tutorial aims to guide researchers and practitioners in navigating the complex deep learning (DL) landscape, focusing on data management, training methodologies, optimization strategies, and deployment techniques. It highlights open-source libraries like the OpenVINO toolkit, OpenVINO Training eXtensions (OTX), and Neural Network Compression Frameworks (NNCF) in streamlining DL development. The tutorial covers how OTX 2.0 simplifies the DL ecosystem (Computer Vision) by integrating various frameworks and ensuring a consistent experience across different platforms (MMLab, Lightning, or Anomalib). It also demonstrates how to fine-tune generative AI models, specifically Stable Diffusion SD with LoRA, and the benefits of customized models in reducing latency and enhancing efficiency. The tutorial explores fine-tuning visual prompting tasks, including Segment Anything Model (SAM). It explains how to fine-tune a SD model with custom data using multiple acceleration methods, and how to deploy the fine-tuned model using OpenVINO Transformation Passes API. Lastly, the tutorial focuses on model optimization capabilities for the inference phase, with the OpenVINO toolkit and OTX library integrating with NNCF to refine neural networks and improve inference speed, especially on edge devices with limited resources. The tutorial includes demos showcasing how OpenVINO runtime API enables real-time inference on various devices.
Bio s:2nd Workshop on Compositional 3D Vision Tue 18 Jun 08:30 a.m.
Workshop: Synthetic Data for Computer Vision Tue 18 Jun 08:30 a.m.
The 3rd Explainable AI for Computer Vision (XAI4CV) Workshop Tue 18 Jun 08:30 a.m.
The 5th Omnidirectional Computer Vision Workshop Tue 18 Jun 08:30 a.m.
7th International Workshop on Visual Odometry and Computer Vision Applications Based on Location Clues Tue 18 Jun 08:30 a.m.
Workshop: Visual Perception via Learning in an Open World Tue 18 Jun 08:30 a.m.
5th Workshop on Continual Learning in Computer Vision (CLVISION) Tue 18 Jun 08:30 a.m.
Workshop: Equivariant Vision: From Theory to Practice Tue 18 Jun 08:30 a.m.
Tutorial: Hsin-Ying Lee · Peiye Zhuang · Chaoyang Wang
3D/4D Generation and Modeling with Generative Priors
In today's metaverse, where the digital and physical worlds blend seamlessly, capturing, representing, and analyzing 3D structures is vital. Advances in 3D and 4D tech have revolutionized gaming, AR, and VR, offering immersive experiences. 3D modeling bridges reality and virtuality, enabling realistic simulations and AR overlays. Adding time enhances experiences with lifelike animations and object tracking, shaping digital interactions.
Traditionally, 3D generation involved directly manipulating data, evolving alongside 2D techniques. Recent breakthroughs in 2D diffusion models have enhanced 3D tasks using large-scale image datasets. Methods like Score Distillation Sampling improve quality. However, biases in 2D data and limited 3D info pose challenges.
Generating 3D scenes and reducing biases in 2D data for realistic synthesis are ongoing challenges. Our tutorial explores techniques for diverse scenes and realism, including 3D/4D reconstruction from images and videos. Attendees learn about various generation methods, from 3D data training to leveraging 2D models, gaining a deep understanding of modern 3D modeling.
In summary, our tutorial covers the breadth of 3D/4D generation, from basics to the latest. By tackling scene-level complexities and using 2D data for realism, attendees gain insight into the evolving 3D modeling landscape in the metaverse.
Bio s:Workshop: Data-Driven Autonomous Driving Simulation (DDASD) Tue 18 Jun 08:30 a.m.
2nd Workshop on Generative Models for Computer Vision Tue 18 Jun 08:30 a.m.
Workshop on Human Motion Generation Tue 18 Jun 08:30 a.m.
IEEE International Workshop on Computational Cameras and Displays Tue 18 Jun 08:45 a.m.
8th Workshop on Media Forensics Tue 18 Jun 08:45 a.m.
FGVC11: 11th Workshop on Fine-grained Visual Categorization Tue 18 Jun 08:45 a.m.
It may be tempting to think that image classification is a solved problem. However, one only needs to look at the poor performance of existing techniques in domains with limited training data and highly similar categories to see that this is not the case. In particular, fine-grained categorization, e.g., the precise differentiation between similar plant or animal species, disease of the retina, architectural styles, etc., is an extremely challenging problem, pushing the limits of both human and machine performance. In these domains, expert knowledge is typically required, and the question that must be addressed is how we can develop artificial systems that can efficiently discriminate between large numbers of highly similar visual concepts.
The 11th Workshop on Fine-Grained Visual Categorization (FGVC11) will explore topics related to supervised learning, self-supervised learning, semi-supervised learning, vision and language, matching, localization, domain adaptation, transfer learning, few-shot learning, machine teaching, multimodal learning (e.g., audio and video), 3D-vision, crowd-sourcing, image captioning and generation, out-of-distribution detection, anomaly detection, open-set recognition, human-in-the-loop learning, and taxonomic prediction, all through the lens of fine-grained understanding. Hence, the relevant topics are neither restricted to vision nor categorization.
Our workshop is structured around five main components:
(i) invited talks from world-renowned computer vision experts,
(ii) invited talks from experts in application domains (e.g., medical science and ecology),
(iii) interactive discussions during poster and panel sessions,
(iv) novel fine-grained challenges that are hosted as part of the workshop, and
(v) peer-reviewed extended abstract paper submissions.
We aim to stimulate debate and to expose the wider computer vision community to new and challenging problems in areas that have the potential for large societal impact but do not traditionally receive a significant amount of exposure at other CVPR workshops.
Workshop: Towards 3D Foundation Models: Progress and Prospects Tue 18 Jun 08:50 a.m.
The 5th Annual Embodied AI Workshop Tue 18 Jun 08:50 a.m.
Workshop: ScanNet++ Novel View Synthesis and 3D Semantic Understanding Challenge Tue 18 Jun 08:50 a.m.
The Sixth Workshop on Deep Learning for Geometric Computing (DLGC 2024) Tue 18 Jun 09:00 a.m.
Workshop: 7th MUltimodal Learning and Applications Tue 18 Jun 09:00 a.m.
Workshop: New frontiers for zero-shot Image Captioning Evaluation (NICE) Tue 18 Jun 09:00 a.m.
Tutorial: Xiaoyang Wu · Hengshuang Zhao · Fuxin Li · Zhijian Liu
All You Need To Know About Point Cloud Understanding
Unstructured point clouds serve as a sparse representation of the 3D world, playing pivotal roles in 3D perception, generation, autonomous driving, virtual/augmented reality, and robotics. Despite their significance, there lacks a comprehensive resource covering state-of-the-art approaches and engineering nuances in deep point cloud networks. This tutorial aims to fill this gap by offering an comprehensive exploration of the subject. It features lectures that progress from classical point cloud backbones to state-of-the-art point transformers, large-scale 3D representation learning (including pre-training technologies), efficient libraries for sparse systems, and diverse applications for deep point cloud networks. Participants will acquire systematic and practical knowledge on managing and extracting robust deep feature representations from point cloud data. They'll also learn to make informed decisions regarding model architectures and data structures when dealing with point cloud data. Armed with these skills, attendees will be well-equipped to comprehend and leverage these models in real-world applications across various fields, including autonomous driving, embodied AI, and other domains grappling with sparse data in low-dimensional Euclidean spaces.
Bio s:Tutorial: Amir Zamir · Andrei Atanov · Andrew Spielberg
Computational Design of Diverse Morphologies and Sensors for Vision and Robotics
Animals exhibit a wide variety of morphologies and sensors, believed to have appeared through billions of years of evolution. Common examples relevant to vision include differences in pupil shapes, the positioning of eyes, various types of eyes, and a varying level of multimodality across animals. Such adaptations are hypothesized to be instances of the so-called Ecological Theory, which posits a strong connection between the specifics of vision and the environment surrounding the agent, its objectives, and its body. How can we replicate this diversity and achieve adaptive design in robotics and vision systems?
In this tutorial, we discuss I) alternative forms of visual sensors that can be useful for real-world robots and II) computational approaches to robot and vision design that can achieve the goal of adaptive design automatically, effectively, and efficiently. The tutorial covers topics in sensing, control, simulation, optimization, and learning-based design for various rigid and soft robots and visual sensors. The material is drawn from state-of-the-art breakthroughs in the field and insights from other disciplines.
This material is accessible to individuals of all backgrounds and levels of expertise.
Bio s:Workshop: Safe Artificial Intelligence for All Domains (SAIAD) Tue 18 Jun 09:00 a.m.
4th Workshop and Challenge on Computer Vision in the Built Environment for the Design, Construction, and Operation of Buildings Tue 18 Jun 09:00 a.m.
Tutorial: Raquel Urtasun · Sergio Casas · Abbas Sadat · Sivabalan Manivasagam · Ioan Andrei Bârsan
All You Need to Know about Self-Driving
A full day tutorial covering all aspects of autonomous driving. This tutorial will provide the necessary background for understanding the different tasks and associated challenges, the different sensors and data sources one can use and how to exploit them, as well as how to formulate the relevant algorithmic problems such that efficient learning and inference is possible. We will first introduce the self-driving problem setting and a broad range of existing solutions, both top-down from a high-level perspective, as well as bottom-up from technological and algorithmic points of view. We will then extrapolate from the state of the art and discuss where the challenges and open problems are, and where we need to head towards to provide a scalable, safe and affordable self-driving solution for the future.
Since last year’s instance (https://waabi.ai/cvpr-2023/), countless new and promising avenues of research have started gaining traction, and we have updated our tutorial accordingly. To name a few example, this includes topics like occupancy forecasting, self-supervised learning, foundation models, the rise of Gaussian Splatting and diffusion models for simulation as well as the study of closed-loop vs. open-loop evaluation.
Bio s:Tutorial: Li Chen · Andreas Geiger · Huijie Wang · Jiajie Xu
Towards Building AGI in Autonomy and Robotics
In this tutorial, we explore the intersection of AGI technologies and the advancement of autonomous systems, specifically in the field of robotics. We invite participants to embark on an investigative journey that covers essential concepts, frameworks, and challenges. Through discussion, we aim to shed light on the crucial role of fundamental models in enhancing the cognitive abilities of autonomous agents. Through cooperation, we aim to chart a path for the future of robotics, where the integration of AGI enables autonomous systems to push the limits of their capabilities and intelligence, ushering in a new era of intelligent autonomy.
Bio s:L3D-IVU: 3rd Workshop on Learning with Limited Labelled Data for Image and Video Understanding Tue 18 Jun 09:00 a.m.
Workshop: Vision and Language for Autonomous Driving and Robotics (VLADR) Tue 18 Jun 09:00 a.m.
Tutorial: Qing Qu · Zhihui Zhu · Yuqian Zhang · Yi Ma · Sam Buchanan · Beidi Chen · Mojan Javaheripi · Liyue Shen · Zhangyang Wang
Learning Deep Low-dimensional Models from High-Dimensional Data: From Theory to Practice
Over the past decade, the advent of machine learning and large-scale computing has immeasurably changed the ways we process, interpret, and predict with data in imaging and computer vision. The “traditional” approach to algorithm design, based around parametric models for specific structures of signals and measurements—say sparse and low-rank models—and the associated optimization toolkit, is now significantly enriched with data-driven learning-based techniques, where large-scale networks are pre-trained and then adapted to a variety of specific tasks. Nevertheless, the successes of both modern data-driven and classic model-based paradigms rely crucially on correctly identifying the low-dimensional structures present in real-world data, to the extent that we see the roles of learning and compression of data processing algorithms—whether explicit or implicit, as with deep networks—as inextricably linked.
As such, this tutorial provides a timely tutorial that uniquely bridges low-dimensional models with deep learning in imaging and vision. This tutorial will show how: 1. Low-dimensional models and principles provide a valuable lens for formulating problems and understanding the behavior of modern deep models in imaging and computer vision; 2. How ideas from low-dimensional models can provide valuable guidance for designing new parameter efficient, robust, and interpretable deep learning models for computer vision problems in practice.
Bio s:Tutorial: Wenjin Wang · Daniel Mcduff · Xuyu Wang
Contactless AI Healthcare using Cameras and Wireless Sensors
Understanding people and extracting health-related metrics is an emerging research topic in computer vision that has grown rapidly recently. Without the need of any physical contact of the human body, cameras have been used to measure vital signs remotely (e.g. heart rate, heart rate variability, respiration rate, blood oxygenation saturation, pulse transit time, body temperature, etc.) from an image sequence of the skin or body, which leads to contactless, continuous and comfortable heath monitoring. The use of cameras also enables the measurement of human behaviors/activities and high-level visual semantic/contextual information leveraging computer vision and machine learning techniques. Understanding of the environment around the people is also a unique advantage of cameras compared to the contact bio-sensors (e.g., wearables), which facilitates better understanding of human and scene for health monitoring. In addition to camera based approach, Radio Frequency (RF) based methods for health monitoring have also been proposed, using Radar, WiFi, RFID, and acoustic signals. The contactless monitoring of camera and RF will bring a rich set of compelling healthcare applications that directly improve upon contact-based monitoring solutions and improve people’s care experience and quality of life, called “AI health monitoring”. In this tutorial, we will give an overview of recent works in this emerging direction.
Bio s:Workshop: Social Presence with Codec Avatars Tue 18 Jun 09:30 a.m.
The First Workshop on the Evaluation of Generative Foundation Models Tue 18 Jun 01:00 p.m.
Workshop: Implicit Neural Representation for Vision Tue 18 Jun 01:00 p.m.
Tutorial: Long Chen · Oleg Sinavski · Fergal Cotter · Vassia Simaiaki · Elahe Arani · Gianluca Corrado · Nikhil Mohan · Jamie Shotton
End-to-End Autonomy: A New Era of Self-Driving
A comprehensive half-day tutorial focused on End-to-End Autonomous Driving (E2EAD), reflecting the significant shift in focus towards this approach within both industry and academia. Traditional modular approaches in autonomous driving, while effective in specific contexts, often struggle with scalability, long-tail scenarios, and compounding errors from different modules, thereby paving the way for the end-to-end paradigm. This tutorial aims to dissect the complexities and nuances of end-to-end autonomy, covering theoretical foundations, practical implementations and validations, and future directions of this evolving technology.A comprehensive half-day tutorial focused on End-to-End Autonomous Driving (E2EAD), reflecting the significant shift in focus towards this approach within both industry and academia. Traditional modular approaches in autonomous driving, while effective in specific contexts, often struggle with scalability, long-tail scenarios, and compounding errors from different modules, thereby paving the way for the end-to-end paradigm. This tutorial aims to dissect the complexities and nuances of end-to-end autonomy, covering theoretical foundations, practical implementations and validations, and future directions of this evolving technology.
Bio s: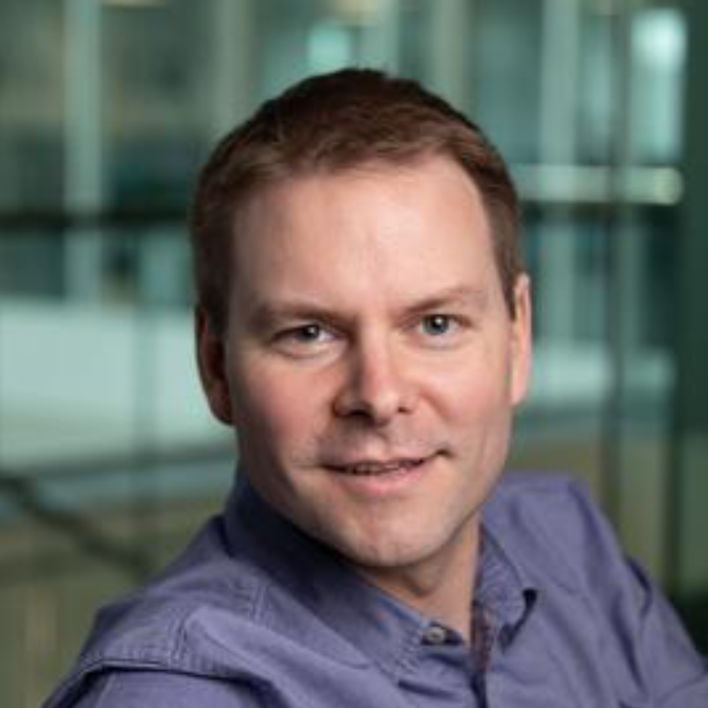
6th Workshop and Competition on Affective Behavior Analysis in-the-wild Tue 18 Jun 01:30 p.m.
Embedded Vision Workshop Tue 18 Jun 01:30 p.m.
The Sixth Workshop on Precognition: Seeing through the Future Tue 18 Jun 01:30 p.m.
Tutorial: Hao Fei · Yuan Yao · Ao Zhang · Haotian Liu · Fuxiao Liu · Zhuosheng Zhang · Shuicheng Yan
From Multimodal LLM to Human-level AI: Modality, Instruction, Reasoning and Beyond
Artificial intelligence (AI) encompasses knowledge acquisition and real-world grounding across various modalities. As a multidisciplinary research field, multimodal large language models (MLLMs) have recently garnered growing interest in both academia and industry, showing an unprecedented trend to achieve human-level AI via MLLMs. These large models offer an effective vehicle for understanding, reasoning, and planning by integrating and modeling diverse information modalities, including language, visual, auditory, and sensory data. This tutorial aims to deliver a comprehensive review of cutting-edge research in MLLMs, focusing on three key areas: MLLM architecture design, instructional learning, and multimodal reasoning of MLLMs. We will explore technical advancements, synthesize key challenges, and discuss potential avenues for future research. All the resources and materials will be made available online: https://mllm2024.github. io/CVPR2024
Bio s:

Workshop: (3rd) Monocular Depth Estimation Challenge Tue 18 Jun 01:30 p.m.
Workshop: Learning from Procedural Videos and Language: What is Next? Tue 18 Jun 01:30 p.m.
Workshop: New Trends in Multimodal Human Action Perception, Understanding and Generation Tue 18 Jun 01:30 p.m.
1st Workshop on Neural Volumetric Video Tue 18 Jun 01:30 p.m.
Tutorial: Maying Shen · Danny Yin · Jason Clemons · Pavlo Molchanov · Jan Kautz · Jose M. Alvarez
Full-Stack, GPU-based Acceleration of Deep Learning
This tutorial focuses on describing techniques to allow deep learning practitioners to accelerate the training and inference of large deep networks while also reducing memory requirements across a spectrum of off-the-shelf hardware for important applications such as autonomous driving and large language models. Topics include, but are not limited to: - Deep learning specialized hardware overview. We review the architecture of the most used deep learning acceleration hardware, including the main computational processors and memory modules. We will also cover aspects of algorithmic intensity and an overview of theoretical aspects of computing.
Best practices for acceleration. We provide an overview of best practices for designing efficient neural networks including channel number selection, compute heavy operations, or reduction operations among others.
Existing tools for model acceleration. In this part we will focus on existing tools to accelerate a trained neural network on GPU devices. We will particularly discuss operation folding, TensorRT, ONNX graph optimization, sparsity.
Foundation models. Here we will focus on best practices for training and deploying foundation models efficiently.
Research overview of recent techniques. In the last part, we will focus on recent advanced techniques for post training model optimization including pruning, quantization, model distillation or NAS among others.
OpenSUN3D: 2nd Workshop on Open-Vocabulary 3D Scene Understanding Tue 18 Jun 01:30 p.m.
5th Workshop on Robot Visual Perception in Human Crowded Environments Tue 18 Jun 01:30 p.m.
20th Workshop on Perception Beyond the Visible Spectrum Tue 18 Jun 01:30 p.m.
Workshop: Representation Learning with Very Limited Images: Zero-shot, Unsupervised, and Synthetic Learning in the Era of Big Models Tue 18 Jun 01:30 p.m.
Workshop: EgoMotion: Egocentric Body Motion Tracking, Synthesis and Action Recognition Tue 18 Jun 01:30 p.m.
Workshop: AVA: Accessibility, Vision and Autonomy Meet Tue 18 Jun 01:30 p.m.
The goal of this workshop is to gather researchers, students, and advocates who work at the intersection of accessibility, computer vision, and autonomous and intelligent systems. In particular, we plan to use the workshop to identify challenges and pursue solutions for the current lack of shared and principled development tools for vision-based accessibility systems. For instance, there is a general lack of vision-based benchmarks and methods relevant to accessibility (e.g., people using mobility aids are currently mostly absent from large-scale datasets in pedestrian detection). Towards building a community of accessibility-oriented research in computer vision conferences, we also introduce a large-scale fine-grained computer vision challenge. The challenge involves visual recognition tasks relevant to individuals with disabilities. We aim to use the challenge to uncover research opportunities and spark the interest of computer vision and AI researchers working on more robust and broadly usable visual reasoning models in the future. An interdisciplinary panel of speakers will further provide an opportunity for fostering a mutual discussion between accessibility, computer vision, and robotics researchers and practitioners.
Tutorial: Mike Zheng Shou · Jay Zhangjie Wu · Deepti Ghadiyaram
Diffusion-based Video Generative Models
In the past year, the landscape of video generation has transformed dramatically, achieving remarkable strides from rudimentary outputs to strikingly realistic videos. Central to this evolution are diffusion models, which have become a cornerstone technology in pushing the boundaries of what's possible in video generation. This tutorial will delve into the critical role of diffusion models in video generation and modeling.
Participants will engage in a deep dive into the broad spectrum of topics related to video generative models. We will start with the foundational elements, including the core principles of video foundation models. The session will then extend to explore specific applications such as image-to-video animation, video editing, and motion customization. A significant focus will also be placed on the evaluation of video diffusion models, as well as on safety technologies to mitigate the potential risks of using these models.
Attendees will leave this tutorial with a comprehensive understanding of both fundamental techniques and the cutting-edge advancements in diffusion-based video modeling, fully equipped to navigate and contribute to the rapidly evolving field in the GenAI era.
Bio s:Tutorial: Zhiqian Chen · Lei Zhang · Liang Zhao
Unifying Graph Neural Networks across Spatial and Spectral Domains
Over recent years, Graph Neural Networks (GNNs) have garnered significant attention. However, the proliferation of diverse GNN models, underpinned by various theoretical approaches, complicates the process of model selection, as they are not readily comprehensible within a uniform framework. Specifically, early GNNs were implemented using spectral theory, while others were developed based on spatial theory . This divergence between spectral and spatial methodologies renders direct comparisons challenging. Moreover, the multitude of models within each domain further complicates the evaluation of their respective strengths and weaknesses.
In this half-day tutorial, we examine the state-of-the-art in GNNs and introduce a comprehensive framework that bridges the spatial and spectral domains, elucidating their complex interrelationship. This emphasis on a comprehensive framework enhances our understanding of GNN operations. The tutorial’s objective is to explore the interplay between key paradigms, such as spatial and spectral-based methods, through a synthesis of spectral graph theory and approximation theory. We provide an in-depth analysis of the latest research developments in GNNs in this tutorial, including discussions on emerging issues like over-smoothing. A range of well-established GNN models will be utilized to illustrate the universality of our proposed framework.
Bio s: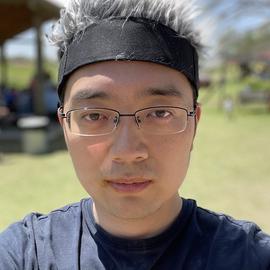